Imagine a classroom in the near future: A teacher uses an AI-powered planner to assign homework, while students prompt AI assistants to complete it for them. The result? Tech-filled classrooms but the learners and teachers are disconnected from the process, and curiosity takes a back seat. This isn’t a warning against AI, but a reflection of what happens when technology is poorly integrated into education.
How can we avoid this trap and design education that fosters authentic learning in a largely AI-dominated world? The problem isn’t the technology itself, but its superficial placement within existing systems. Far from being just another tool for convenience, generative AI is redefining what we learn and how we learn. This shift raises deeper questions: What knowledge is worth mastering in an AI-driven world, and how do we ensure meaningful learning? To understand this change, we need to examine two critical axes:
What We Learn
- The value of expertise when AI can generate expert-level content.
- The meaning of being “educated” in a world of instant information.
- The balance between information vs knowledge assimilation.
How We Learn
- The transformation of personalization when AI adapts to individual learning pathways and contexts.
- The role of AI as a learning partner beyond its function as a content delivery tool.
- The shift from traditional assessment to AI-enabled continuous feedback systems.
Focusing on “how we learn” before clarifying “what we learn” could lead to digitizing outdated content and practices. As Justin Reich highlights in Failure to Disrupt, many educational innovations have simply increased access to content without transforming pedagogy.
Learning From Past Technological Disruptions
History shows us that technological change reshapes education in ways we often fail to anticipate. The printing press didn’t just replace hand-copied manuscripts – it democratized knowledge and sparked mass literacy. When calculators entered classrooms, many feared students would lose mental math skills. Instead, calculators freed learners to engage in higher-order problem-solving.
Technological disruption in education often follows a familiar pattern: innovations improve access to content without transforming the learning process. For example, Massive Open Online Courses (MOOCs) greatly expanded access to educational materials but largely reproduced traditional lecture-based teaching models.
The current wave of AI integration risks repeating the same mistake. Companies are adding AI chatbots to content delivery systems without rethinking what students should be learning in an AI-enabled world.
For education to thrive in an AI-driven world, we must rethink traditional notions of knowledge, mastery, and expertise. The following key shifts are essential to prepare learners for a future where adaptability, creativity, and collaboration with AI define success.
Compounding this issue is the accelerating pace of technological disruption. In recent years, technology cycles have become shorter, with innovations emerging faster than ever. Traditional K-12 competency frameworks, which are often static and slow to evolve, struggle to keep pace with these changes.
For education to thrive in an AI-driven world, we must rethink traditional notions of knowledge, mastery, and expertise. The following key shifts are essential to prepare learners for a future where adaptability, creativity, and collaboration with AI define success.
Let’s unpack what needs to shift:
Rethinking Mastery In The Age Of AI
Traditionally, education has focused on acquiring subject-specific knowledge, often assessed through memorization and recall. But in a world where AI can instantly generate expert-level content, mastery can no longer be defined by static knowledge. As Ilkka Tuomi argues in his paper Beyond Mastery, mastery now means critical synthesis, reasoning, and the ability to apply knowledge creatively in new contexts. He wrote: “When knowledge becomes instantly accessible and expertise can be generated on demand, we must reconsider what it means to be educated.”
We must ask: What knowledge is timeless, and what knowledge is context-specific?
- Foundational knowledge (literacy, numeracy, and scientific reasoning) remains essential, but it should be treated as a gateway to problem-solving and exploration, not as an endpoint.
- Interdisciplinary knowledge, such as systems thinking, ethics, and societal impact, is increasingly valuable. Students must understand how disciplines interact to address complex challenges like climate change or technological ethics.
Traditionally, education has focused on acquiring subject-specific knowledge, often assessed through memorization and recall. But in a world where AI can instantly generate expert-level content, mastery can no longer be defined by static knowledge.
This shift aligns with Bloom’s Taxonomy, which prioritizes progressing from remembering and understanding to higher-order thinking such as analyzing, evaluating, and creating. Generative AI accelerates this shift by automating lower-order tasks, freeing students to focus on creativity, critical evaluation, and problem-solving.
A good example of this shift is found in TUMO’s AI-driven learning centers, where students take control of their learning paths through interdisciplinary projects, exploring topics like digital media and environmental sustainability. This hands-on approach nurtures the skills students need to navigate real-world complexity and evolving knowledge landscapes.
Redefining Expertise In An AI-Enabled World
Generative AI doesn’t eliminate the need for expertise – it redefines it. Historically, expertise has been guarded by institutions, rigorous training programs, and years of deliberate practice. AI disrupts this model by making specialized knowledge accessible to anyone with an internet connection. Tools like ChatGPT, GitHub Copilot, and AI-driven platforms such as Khan Academy or Coursera enable novices to bypass traditional gatekeepers.
- A hobbyist coder can now build complex apps using AI-generated code.
- A farmer in a remote region can access precision agriculture tools powered by AI, optimizing crop yields without formal agronomy training.
The result is a shift from “knowing” to “connecting.” When there is an abundance of information available the crucial skill is to connect various pieces and make meaning of it. As AI absorbs vast amounts of information, human expertise may pivot from possessing knowledge to curating and contextualizing it. Experts will increasingly act as guides who filter AI-generated insights, ensuring relevance and accuracy. This mirrors the Renaissance ideal of the “polymath” but with AI as a collaborator rather than a competitor.
Generative AI doesn’t eliminate the need for expertise - it redefines it. Historically, expertise has been guarded by institutions, rigorous training programs, and years of deliberate practice. AI disrupts this model by making specialized knowledge accessible to anyone with an internet connection.
Moreover, traditional models of expertise, which emphasize mastering static knowledge, are no longer sufficient. As VanLehn found in a meta-analysis of intelligent tutoring systems, these systems can match human tutors in delivering learning gains but haven’t transformed how students develop adaptive expertise i.e. the ability to apply knowledge flexibly to novel situations.
Programs like Synthesis School, originally designed for the children of SpaceX employees, immerse students in dynamic simulations that mimic real-world challenges. Students must continuously adjust their problem-solving strategies as conditions change, demonstrating how adaptive expertise enables flexible thinking and better prepares them for unpredictable environments.
Developing Transdisciplinary Skills
To complement adaptive expertise, education must focus on content-agnostic, transferable skills that enable students to navigate a rapidly changing world:
- Framing questions effectively: As seen in platforms like hitwit.ai, iterative prompting exercises train students to ask meaningful questions, critically evaluate AI responses, and refine their problem-solving processes.
- Metacognitive mastery: Understanding how to learn, as emphasized by Azevedo and Hadwin prepares students to be lifelong learners capable of adapting to new technologies and contexts.
- Synthesis and decision-making: When students engage with teachable agents like Betty’s Brain, they externalize their understanding by teaching a virtual agent, helping them connect concepts and reason through complex systems.
This focus is essential because content creation itself is no longer a higher-order learning outcome. In an AI-enabled world, students must critically evaluate, synthesize, and improve upon AI-generated outputs, equipping themselves to thrive in roles where machines and humans collaborate.
In an AI-enabled world, students must critically evaluate, synthesize, and improve upon AI-generated outputs, equipping themselves to thrive in roles where machines and humans collaborate.
Conclusion: Don’t Let The Rocket Land On A Nearby Hill
As educational frameworks evolve to address rapid technological change, ed tech products and programs must also shift their focus. We cannot rely solely on evolving competency models — ed tech needs to be prepared to drive this transformation by embedding adaptability, critical thinking, and creativity into core learning experiences.
If you’re an aspiring “edu-preneur” or a learning engineer, resist the temptation to create superficial AI products like chatbots layered onto existing content delivery systems. Instead, start by asking what is worth learning and build solutions that foster deep engagement and transferable skills.
Because without that pause, your innovation might be like a rocket landing on a nearby hill – impressive in effort, but far from its potential destination. To truly revolutionize learning, we must aim higher. By aligning AI integration with meaningful learning objectives, we can ensure students are prepared to thrive in an ever-changing world
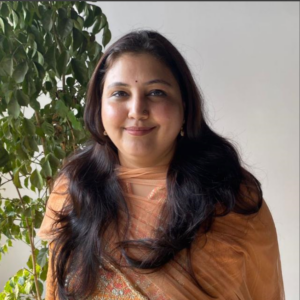
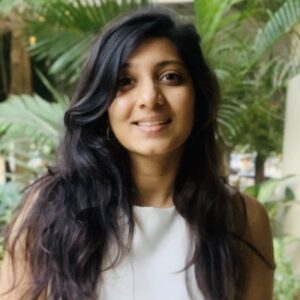