As artificial intelligence transforms not just education but the entire landscape of work and society, educators face a pivotal moment. The traditional debate about whether AI will replace teachers – or should be resisted by them – misses a crucial point: the future belongs to those who can effectively partner with AI. These partnerships may range from enhancing productivity in familiar tasks to enabling entirely new capabilities that were previously impossible.
The report by the Bipartisan House Task Force on Artificial Intelligence underscores this imperative. Among its key findings:
- “AI is increasingly used in the workplace by both employers and employees: It is likely that workers will increasingly work with or alongside AI systems, which will require pathways to upskill and AI-enabled workforce” (p.106).
- “AI adoption in America requires AI literacy: A lack of understanding of AI could lead the public to avoid AI products, missing out on productivity-enhancing or quality-of-life-improving uses of the technology” (p. 106).
- “K-12 educators need resources to promote AI literacy: To achieve AI literacy and education for students, teachers need knowledge of AI technology, including AI training on using AI in the classroom” (p.106).
The implications of these findings are profound. Some AI tools simply make existing tasks more efficient, while others enable entirely new possibilities by handling complexity beyond human cognitive limits. Meeting the coming challenge isn’t just about changing how to teach – it’s about understanding and leveraging both types of AI partnerships to transform education while preparing students for a world where human-AI collaboration will be fundamental to success.
Understanding Human-AI Partnerships Through Distributed Cognition
The concept of distributed cognition, pioneered by cognitive scientist Edwin Hutchins offers a framework for understanding these partnerships. Through his groundbreaking study of naval navigation teams, Hutchins demonstrated how cognitive processes extend beyond individual minds to encompass tools, environments, and other people. His work showed that complex cognitive tasks are achieved through the interaction of multiple components, each contributing unique capabilities.
While distributed cognition has always been part of human learning and work, AI introduces new possibilities for these partnerships. To understand how distributed cognition works in educational settings, consider Personal Assistant for Learning (PAL), an AI system currently in development for early math learning. Set to begin pilots in January, PAL’s design exemplifies the distributed cognition framework. Rather than attempting to teach children directly, PAL is designed to create cognitive partnerships with teachers and caregivers.
In this planned partnership, PAL’s AI system and adult educators create a dynamic learning ecosystem. PAL contributes sophisticated knowledge modeling of early mathematics concepts and their relationships, using predictive analytics to identify activities at the leading edge of each child’s learning capabilities—their Zone of Proximal Development, or ZPD. Teachers and caregivers provide crucial data about children’s engagement with activities and bring essential expertise in instruction. Given documented gaps in early math knowledge among both teachers and parents, PAL also supports adults with detailed information about mathematical concept relationships and effective instructional strategies.
Teachers and caregivers provide crucial data about children's engagement with activities and bring essential expertise in instruction. Given documented gaps in early math knowledge among both teachers and parents, PAL also supports adults with detailed information about mathematical concept relationships and effective instructional strategies.
The partnership functions through continuous interaction: Adults implement learning activities with children and provide feedback about the child’s performance, which PAL then uses to refine its understanding of each child’s ZPD. Using its comprehensive knowledge model and sophisticated algorithms, PAL then predicts which concepts and activities will best support the child’s next learning steps. Throughout this process, adults make real-time instructional decisions, adapting activities based on their understanding of each child’s needs and context. In addition, PAL also provides important “just-in-time” information to the adults designed to increase their expertise. These bite-sized “tips” include insights about learning science, cognitive development, and child development theories underpinning the suggested activities.
Why does this partnership work, and what makes it a true distributed cognitive system? The key lies in how each partner’s capabilities address the other’s limitations. The AI manages complexity that humans cannot – tracking hundreds (and eventually thousands) of mathematical concepts, their relationships, and optimal learning sequences across multiple children simultaneously. Meanwhile, the human partners provide what AI cannot—meaningful engagement with young children, interpretation of subtle learning nuances, and dynamic instructional adjustments. Neither partner alone could achieve optimal learning outcomes—the AI cannot engage developmentally with children, while even skilled teachers cannot track and analyze the intricate web of learning progressions at scale. The power lies in how these complementary capabilities create a system greater than the sum of its parts.
The Evolution of Teaching Practice: Why Current Models Fall Short
Both current teaching practices and educational technology developers often approach AI narrowly – either as a mere productivity tool or as a potential replacement for teachers. These limited perspectives miss the transformative potential of a true human-AI partnership. Many teachers still work in isolation, carrying the full cognitive load of tracking student progress, designing interventions, and making instructional decisions for dozens of students across multiple subjects and skills.
This traditional teaching model becomes increasingly unsustainable as researchers better understand the complexity of learning progressions and the need for personalization—especially for the youngest learners. Some teaching challenges can be addressed through productivity-enhancing AI tools, but others—like responding to detailed learning trajectories and in-the-moment learning needs of multiple students across hundreds of mathematical concepts—require AI partnerships that extend human cognitive capabilities. Yet many professional development programs still focus on having teachers manage this complexity alone, just with newer strategies or productivity tools.
This misalignment between current practice and future needs limits the potential impact of both human expertise and AI capabilities by keeping them separate rather than synergistic.
This misalignment between current practice and future needs limits the potential impact of both human expertise and AI capabilities by keeping them separate rather than synergistic. It perpetuates a model of teaching that can’t scale to meet the increasing demands for personalized learning. Teachers need to develop new expertise in creating and managing distributed cognitive partnerships – understanding both when AI can enhance productivity and when it can enable entirely new teaching capabilities.
From Theory to Practice: Reimagining Teacher Expertise
Moving toward a distributed cognition model requires fundamentally reimagining teacher expertise. While PAL illustrates how AI can extend human cognitive capabilities in early mathematics, the framework applies broadly across education. Consider current AI writing assistants – some simply enhance productivity through automated grading, but more sophisticated implementations create true cognitive partnerships where AI handles complex language analysis while teachers focus on developing student voice and critical thinking.
This shift to orchestrating human-AI partnerships demands new forms of professional expertise. Teachers need to develop skills in creating effective cognitive systems that leverage both types of AI capabilities. This means knowing when to use AI for productivity enhancement versus capability extension, how to evaluate AI suggestions, and how to integrate AI capabilities with human instruction for optimal learning outcomes. Most importantly, it means understanding how to maintain the dynamic feedback loop between human expertise and AI analysis that makes these partnerships powerful.
Transforming Professional Development for Human-AI Partnership
In this new paradigm, professional development must move beyond traditional “tool training” to develop expertise in orchestrating distributed cognitive systems. This includes:
- Evaluating AI capabilities and limitations within specific educational contexts. Teachers need support in learning to distinguish between AI tools that simply enhance productivity and those that enable entirely new teaching possibilities, developing the same kind of nuanced understanding of AI capabilities that they bring to other aspects of their practice.
- Designing integrated learning experiences that leverage both human and AI strengths. Rather than treating AI tools as add-ons, teachers need to weave them seamlessly into their instructional practice, creating fluid partnerships that transform teaching and learning.
- Making sophisticated decisions about AI integration. This involves developing professional judgment about which aspects of teaching and learning benefit from AI partnership and which are best served by human expertise alone.
Teacher preparation programs will need to transform at a systemic level to support this evolution. Future teachers need opportunities to experience AI partnerships firsthand, developing both theoretical understanding and practical skills through case studies and direct practice with sophisticated AI tools.
School systems should also reimagine how they support teacher growth in this area. One-off workshops should be replaced with ongoing professional learning communities where teachers can experiment with different types of AI partnerships, share insights, and collectively develop best practices. Schools will need to create safe spaces for teachers to innovate and occasionally fail as they develop these new forms of expertise.
Teacher preparation programs will need to transform at a systemic level to support this evolution. Future teachers need opportunities to experience AI partnerships firsthand, developing both theoretical understanding and practical skills through case studies and direct practice with sophisticated AI tools.
Beyond Education: Stakes and Implications
Teachers stand at the forefront of one of society’s most crucial experiments in human-AI partnership. The success or failure of educators in developing effective distributed cognitive systems will have implications far beyond the classroom. As the Bipartisan House Task Force noted, workers across sectors will increasingly work with and alongside AI systems. Education provides a unique testing ground for these partnerships, where the stakes – children’s learning and development – demand thoughtful integration of human expertise and AI capabilities.
The cost of avoiding or misunderstanding these partnerships is significant. Without AI’s capability to manage complex learning progressions, teachers face an impossible choice: either attempt to monitor and personalize learning for dozens of students across hundreds or thousands of concepts and skills (an overwhelming cognitive task), or rely on opaque AI-driven programs that exclude teacher expertise from the decision-making process. This dichotomy fuels teachers’ fears about AI – that they must either burn out trying to do it all or be gradually replaced by AI systems that minimize their role. Yet neither option is acceptable: AI systems without teacher expertise in implementation and relationship-building cannot effectively support learning. The distributed cognition framework offers a better way forward, where AI and human expertise work in genuine partnership rather than in competition. These partnerships create transformative possibilities for personalizing education at scale that go far beyond simple productivity gains.
Teachers stand at the forefront of one of society's most crucial experiments in human-AI partnership. The success or failure of educators in developing effective distributed cognitive systems will have implications far beyond the classroom.
As early adopters of human-AI partnerships, how teachers navigate this transition will likely inform other professions’ approaches to human-AI collaboration. Education offers valuable lessons about balancing automation with human judgment, managing the integration of AI insights into professional practice, and maintaining human relationships while leveraging AI capabilities. Success in educational settings could provide models for healthcare, social services, and other fields where human expertise must be augmented rather than replaced by AI.
As we move forward, the question is not whether to embrace AI partnerships in education, but how to do so thoughtfully and effectively. Teachers who develop expertise in orchestrating these partnerships – knowing when to use AI for productivity enhancement and when to engage in deeper cognitive partnerships – will be better equipped to meet the growing demands of personalized learning. More importantly, they will help shape how society approaches the integration of AI into professional practice, leading the way toward a future where human expertise and AI capabilities work together to enhance learning for all students.
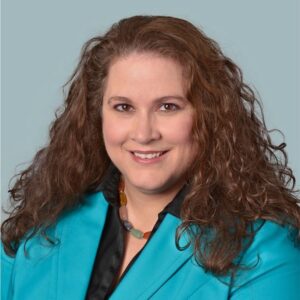