As artificial intelligence (AI) continues to advance at an unprecedented pace, competition among tech giants is intensifying. The rise of DeepSeek and its challenge to leading players like GPT, Claude, and Gemini show that the race for dominance in the AI field has gone global. On the one hand, this competitive acceleration can spell promise for the education field. If the AI arms race yields tremendous growth in the performance of AI agents and large language models (LLMs), their adoption in educational settings can enhance teaching and learning.
On the other hand, how can the education field be sure which tech firm is actually winning the race, and which models are suitable for the classroom? Measuring AI performance requires rigorous evaluation methods that go beyond corporate marketing claims. But that’s where benchmark datasets come in.
Benchmark datasets enable everyone – from everyday consumers to specialists, engineers, and tech CEOs – to assess how various models perform across different domains. Benchmarking provides an objective way to compare a GPT to a DeepSeek, ensuring transparency and accountability in these models’ development. Benchmark datasets are based on widely recognized, available, or standardized tasks for evaluating AI machines.
Our team has been building some benchmarks to create a stronger foundation for assessing how well these models support student learning. For instance, our PERSUADE dataset has become an important tool for benchmarking AI performance in writing evaluation. It is the largest publicly available collection of middle and high school student essays, and each essay was labeled for their various structural and argumentative elements as well as their effectiveness in supporting the student’s argument.
Our benchmarks span other educational domains and challenges, such as managing data privacy and security through the automatic detection and removal of personally identifiable information (PII). Other directions for educational benchmarking include more complex and multi-modal datasets to capture richer learning experiences. For instance, imagine a dataset that combines classroom audio transcripts, student writing, and facial expression data to analyze student motivation and engagement. The ability to use AI to drive insights into student learning behaviors would expand with this kind of benchmark, leading to better tools that can support teachers and students.
Benchmarking provides an objective way to compare a GPT to a DeepSeek, ensuring transparency and accountability in these models’ development. Benchmark datasets are based on widely recognized, available, or standardized tasks for evaluating AI machines.
A Commitment To Open Benchmarking
At The Learning Agency, our commitment to open benchmarking drives our strategy for improving AI in education. We host data science competitions as a key lever for developing and promoting benchmarks. These competitions create open challenges where members of the global machine learning community – whether they specialize in education research or not – can train high-quality and open-source algorithms.
For instance, we partnered with Kaggle last year to run a competition on the task of automatically detecting AI-generated essays. Our benchmark contains both human- and machine-written essays, and we crowdsourced over 10,000 competitors and nearly 100,000 solutions. We learned that open benchmarks, especially when released or promoted through competitions, lead to broader research adoption and attract the attention of major tech companies in addressing educational challenges.
While tech firms release their own reports, data, and documentation to showcase how their models outperform others, they often rely on external benchmarks to track, monitor, and compare performance. The development and release of education benchmarks would hold them accountable for how their models help – or harm – teaching and learning.
We have learned that open benchmarks, especially when released or promoted through competitions, lead to broader research adoption and attract the attention of major tech companies in addressing educational challenges.
We’ve also learned that equity-focused benchmarks play a crucial role in making AI fairer and more inclusive in education. When benchmarks represent historically marginalized populations (e.g., students with special needs, English Language Learners) or include robust metadata on the learner —such as their race, ethnicity, or socioeconomic background—they are more likely to yield equitable AI solutions. AI models learn to predict general patterns but often contain cultural biases. Equity-focused benchmarks play a key role in facilitating efforts to create more responsible AI in educational settings.
Collaboration Is Key
Collaboration is also essential to benchmark development. The Learning Agency team has worked with experts in educational research, technology, and practice to develop our benchmarks. These collaborative, cross-disciplinary efforts help push the boundaries of innovation in AI while ensuring the data reflects real-world educational challenges.
After all, the risks of adopting AI for the classroom are higher due to issues of ethics and fairness. As such, benchmark datasets can serve as an accountability tool. They can promote open, reproducible research by highlighting both the strengths and limitations of AI models, including potential biases in practice.
At The Learning Agency, we are excited to be part of this new wave of open, education-focused benchmarks, but we recognize that there is still a long way to go in making these benchmarks more openly available and accessible. Through collaboration, we can build datasets that span multiple domains, address real-world educational challenges, and shape the future of AI-driven learning.
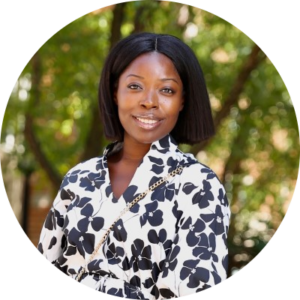
Perpetual Baffour
Research Director