Millions of Americans remain unemployed and navigate a stagnant labor market where even qualified candidates encounter hidden barriers that may hinder their career advancement.
Job seekers and career explorers will need innovative, accessible, and personalized solutions to overcome these challenges, and AI – specifically, large language models (LLMs) – is a promising tool in this regard. LLMs can address the need for personalized, on-demand career guidance, offering quick, accessible, and tailored solutions that could reshape how individuals navigate their professional journeys.
While the possibilities are exciting, challenges remain. Key issues, such as ensuring accurate and fair guidance while maintaining a human-like touch, still need to be addressed. However, AI’s potential to democratize career advice and make it more inclusive is undeniable. With LLMs, career coaching can become more accessible and flexible, catering to a diverse range of job seekers and career stages.
This piece explores the strengths and limitations of AI in career coaching while suggesting future directions for its integration. AI’s potential is clear, but the future of career coaching lies in blending cutting-edge technology with human-centered expertise, evolving to meet the growing demands of today’s workforce.
Strengths of Career Coaching LLMs
Career coaching powered by LLMs can offer convenient and valuable guidance, enabling individuals to take control of their career paths. With the ability to address various concerns and provide real-time feedback, LLMs can be a key resource for those navigating the job market.
One of the benefits of LLM career coaching is its anonymity. Seeking help can be a vulnerable experience. The vulnerability of sharing career struggles, especially when considering a job change or facing challenges at work, can often lead individuals to shy away from asking for advice. LLMs create a safe, comfortable, and anonymous environment to encourage people to take the first step to seek the support they need.
Additionally, LLMs are incredibly convenient. Unlike traditional coaches, they are available 24/7, allowing users to access advice when they need it most, without the need for scheduling. LLMs can simultaneously handle multiple interactions seamlessly and have the ability to communicate in multiple languages, making career advice accessible to a diverse and global audience.
Career coaching powered by LLMs can offer convenient and valuable guidance, enabling individuals to take control of their career paths. With the ability to address various concerns and provide real-time feedback, LLMs can be a key resource for those navigating the job market.
Career advice from LLMs is not only specific but also actionable and measurable. Self-determination theory highlights that people feel most empowered when they can take action that leads to real change. LLMs can foster this sense of autonomy by breaking down complex career goals and suggesting actionable, realistic steps, building users’ competence, and motivating them to stay on course with their career goals.
Moreover, LLMs can evolve over time, adapting to a user’s individual context—whether it’s their current job responsibilities, skillset, or career aspirations. This ability to personalize coaching ensures that users receive advice tailored to their unique needs. As AI technologies continue to improve, LLMs will be able to offer even more refined and insightful guidance, enhancing the user experience.
Limitations of Career Coaching LLMs
Despite these advantages, current LLMs come with notable limitations that impact their effectiveness. From struggling with generating accurate and fair guidance to lacking personal touch and career stage variation. These AI tools face several obstacles to delivering comprehensive career guidance.
One limitation is bias. LLMs rely on past data to generate predictive outputs, but this process can result in biased outcomes that perpetuate systemic social injustices against minority groups. In other words, an LLM might learn to generate responses that discourage certain groups from pursuing specific career fields, further reinforcing existing barriers. As a result, there needs to be a focused effort to expand the demographic representation of career seekers in the training data. Additionally, strategies should be developed to improve outcomes for marginalized populations such as diversifying occupational profiles. For instance, including data on minority physicians’ unique needs and avoiding cultural bias helps an AI career coach support, rather than discourage, underrepresented medical aspirants.
Emotional support can be critical in a career coaching relationship and is another area where LLMs fall short. In certain situations, the emotional intelligence needed to guide a career path may require a human touch that goes beyond the personalization and user context the LLM has been trained to learn. Considering a person’s capabilities, work history, goals, interests, and other factors can lead to more specific and fruitful career guidance. However, LLMs are unlikely to be programmed to empathetically address or inquire about other relevant aspects of the individual in their career path.
Emotional support can be critical in a career coaching relationship and is another area where LLMs fall short. In certain situations, the emotional intelligence needed to guide a career path may require a human touch that goes beyond the personalization and user context the LLM has been trained to learn.
Additionally, LLMs are known to generate inaccurate or misleading information, which can lead users to make poor decisions regarding their career paths. Since LLMs are evaluated primarily based on user feedback rather than the accuracy of the information they provide, users might trust these tools too much, assuming the advice is reliable when it may not be. Many users lack the career coaching or training background to critically evaluate the legitimacy of the answers provided, creating a real risk of harm. To address this, there needs to be an emphasis on improving the accuracy and reliability of AI-driven advice with better evaluation metrics, benchmarks, and feedback systems.
These limitations stem from a common root issue: a lack of quality data and benchmarking tools for AI career coaching. An LLM is only as good as the data it is trained on, and currently, there are limited datasets focused on career paths and ensuring quality advice. This lack of representative data poses a challenge for developing tailored AI solutions that can provide authentic, accurate advice for diverse users. However, LLMs are rapidly evolving, and there are clear growth opportunities.
The Future of Career Coaching LLMs
The limitations of career coaching LLMs don’t imply that these AI tools won’t succeed in the future. On the contrary, these limitations highlight crucial areas where development efforts should be focused to maximize their effectiveness. Rather than seeing these challenges as insurmountable, they should be viewed as opportunities for refinement.
One of the primary areas for improvement is the development of benchmark datasets that are not only demographically representative but also accurately annotated to improve the AI’s understanding of diverse career paths. For example, LLMs help with generic job matchings but are not good at assessing whether an individual is a good cultural fit for a company or whether a job truly aligns with their personal values and lifestyle preferences. Datasets should reflect a wide array of factors, including the user’s demographic background, as well as varied career stages, and individual skills, interests, and goals. With the right data, LLMs can generate more accurate and tailored guidance for users at any point in their career journey, ensuring that no group is left behind.
Datasets should reflect a wide array of factors, including the user’s demographic background, as well as varied career stages, and individual skills, interests, and goals. With the right data, LLMs can generate more accurate and tailored guidance for users at any point in their career journey, ensuring that no group is left behind.
Another promising area for growth is the creation of AI-driven modules that focus on real-world challenges faced by career seekers. For example, LLMs can suggest job titles or general career advice but cannot yet provide a comprehensive and continuously updated analysis of global job markets in real time. A highly tailored career strategy, taking into account future job trends, niche industries, or fast-evolving fields (like AI or cybersecurity) would be a valuable LLM for career explorers. By offering insights into future career directions, AI can help users make more informed career choices.
Interview preparation is another area where LLMs have immense potential. Rather than just offering generic advice, AI could be programmed to simulate job-specific interview questions tailored to the process description. LLMs can simulate interview questions and offer general feedback but often struggle to provide personalized, actionable critiques based on specific interview performance, such as evaluating non-verbal cues, emotional intelligence, or the “flow” of conversation. This would allow users to practice responses that are highly relevant to their career aspirations. In addition, the LLM could provide personalized feedback, helping users refine their answers and improve their overall presentation. Such tools could help job seekers gain confidence and perform better in real job interviews.
Call to Action
While AI in career coaching shows promise, there’s still much work to be done through collective effort. So, where do we begin? One promising direction is increased investment in robust, representative datasets that address all career navigation interests and backgrounds. These datasets can train, evaluate, and benchmark the performance of AI career coaching models. Additionally, continued research on relevant aspects of the career journey—such as goals, aspirations, current skills, and past work history—is essential to supporting the accuracy of these tools. By expanding the scope of career coaching LLMs, we can develop equitable tools that meet the individual needs of users, regardless of their background or career stage.
Redirecting AI resources toward real-world challenges and focusing on developing datasets that support career guidance and thus promote social mobility is crucial for improving LLM-based career coaching. Overall, career coaching LLMs possess great value for those in the job market, but we need to invest in them to unlock their full capability.
To support the development of accurate and equitable career navigation tools that work, CareerNet, a Renaissance Philanthropy partnership with The Learning Agency and CareerVillage.org invested in by the Schultz Family Foundation, will build performance benchmarks for AI platforms for career navigation tasks and spur innovation through developer competitions.
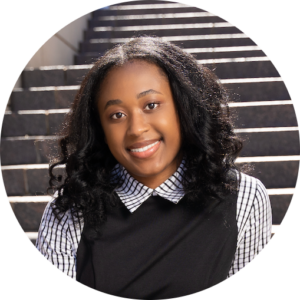
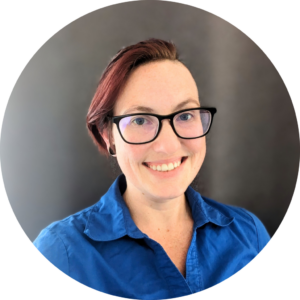