Generative AI (GenAI) tools like ChatGPT, Gemini, and Claude are changing how people find and learn new information. Instead of having to visit multiple websites to get relevant information or find answers to questions, anyone can access GenAI tools that provide well-structured, easy-to-understand answers in seconds. Tools like Perplexity.ai, Google AI Overview, and SearchGPT even provide links to their sources, allowing users to dig deeper to find information that supports the AI response or to verify its accuracy. It’s no surprise, then, that nearly half of U.S. teens and young adults (ages 14–22) were already using GenAI in 2024. That number has probably only gone up since then.
But while GenAI makes accessing information quite convenient, it may come at a cost, especially when it comes to how we think about and learn from the information provided by GenAI tools. To understand and address the potential adverse effects of GenAI use on our thinking and learning, we first need to understand how learning works.
The Thinking Behind Learning
One of the most well-known frameworks in education, Bloom’s Revised Taxonomy, breaks learning down into different levels: starting with remembering and understanding, then moving up to the more complex processes of applying, analyzing, evaluating, and finally, creating. Learning is more than just getting the right answer—it involves actively working with information, questioning it, and using it to build something new.
There’s also a layer of thinking about our own thinking, called metacognition. This includes being aware of what we know (and what we don’t), figuring out the best strategies to learn something, and adjusting these strategies when things don’t work as expected, such as upon realizing that a chosen learning strategy is not working.
Overall, the process of learning can be broken down into three main steps:
- Picking up new information—facts, ideas, or skills—that gives us a base to build on.
- Working with that information by thinking it through, connecting it to what we already know. This step involves engaging in cognitive processes to transform the knowledge we acquired in the first step into a deeper understanding.
- Reflecting on our thinking through metacognition. This means being aware of how well we’re learning and making changes to our approach as needed. A big challenge with effective metacognitive engagement is overcoming the tendency to be overconfident in how much we know.
What Happens When AI Does The ‘Thinking’ For Us?
Now, imagine a student trying to learn programming. They run into a tough problem. Without AI, they might search online, read a few forum threads, watch a video, and piece together their own understanding. In the process, they’d have to put some effort into understanding the information provided in different online resources, apply what they’ve learned to the problem at hand, analyze information from different resources, and evaluate alternative solutions to decide what works best. By engaging in all these different types of thinking processes, the student isn’t just learning more deeply—they are also practicing key cognitive skills like problem-solving, analyzing, and decision-making. And as they keep practicing these skills, they also become more aware of how they learn best, which helps them improve their metacognitive abilities.
But what happens when the same student turns to a GenAI tool to seek answers? They might just ask a chatbot and get a ready-made answer. But, in this process, they may skip over a lot of the effort that leads to deep understanding. As a consequence, the student would also miss out on practicing essential skills such as applying concepts, and analyzing and evaluating solutions. Over time, this kind of “cognitive offloading” could slow down the development of crucial thinking and metacognitive skills, especially for younger students who have not sufficiently developed their thinking abilities.
Evaluating AI-generated content requires a certain level of background knowledge of the topic you are inquiring about, along with a good sense of how confident (or uncertain) you should be about your understanding of the topic. That can be difficult if you are a beginner or if you don’t realize when you might be wrong.
It can be argued that students still need to evaluate whether the AI response is correct. However, that’s easier said than done. Evaluating AI-generated content requires a certain level of background knowledge of the topic you are inquiring about, along with a good sense of how confident (or uncertain) you should be about your understanding of the topic. That can be difficult if you are a beginner or if you don’t realize when you might be wrong.
Why Reflective Thinking Matters More Than Ever
“We do not learn from experience… we learn from reflecting on experience.” – John Dewey
One of the biggest risks with over-relying on GenAI is that it could harm our reflective thinking abilities—the kind of deep, conscious thought that drives deep and effective learning. John Dewey, a prominent philosopher and education reformer, described reflective thinking as thinking that involves carefully evaluating evidence and questioning beliefs. According to Dewey, for this kind of thinking to happen, a few things need to be in place:
- A sense of perplexity, confusion, or doubt that sparks curiosity
- Prior knowledge to build on
- Active, persistent exploration of ideas
- An openness to uncertainty during further exploration
- The ability to connect and evaluate related ideas
Over-relying on GenAI can short-circuit these steps in several ways. First, getting instant, neatly packaged answers may discourage thinking about what one has actually understood, which can take away the opportunity of feeling confused or curious. Next, because GenAI tools are trained to sound confident and agreeable, they may reinforce what we already believe, instead of challenging us to think differently, therefore encouraging confirmation bias. Finally, when GenAI outputs appear to be structured and comprehensive, they can create an illusion of comprehensive understanding, when in reality, people may only achieve a superficial grasp of the underlying concept. This explains why learners may think they’ve learned more from AI tools than they actually have.
When GenAI outputs appear to be structured and comprehensive, they can create an illusion of comprehensive understanding, when in reality, people may only achieve a superficial grasp of the underlying concept. This explains why learners may think they’ve learned more from AI tools than they actually have.
Supporting Student Thinking In The Age Of AI
Now that we’ve seen how GenAI can shortcut important thinking processes, how can educators help students grow as thoughtful, reflective learners even as AI becomes part of everyday learning?
Before exploring this question further, it is important to understand that GenAI itself isn’t inherently good or bad for thinking—it depends on how it is being used and by whom, such as whether it is being used passively or actively. Passive use, like just copying answers or accepting them without further questioning, can weaken our thinking abilities. But if we design learning experiences that encourage active engagement with GenAI, we can use it as a tool to strengthen students’ thinking skills.
One promising approach is to draw on Dewey’s theory of reflective thinking and the conditions he outlined as the prerequisites for it. Using this theory as a framework can guide us in two ways:
- To identify when student-AI interactions are supporting reflective thinking, by checking whether the prerequisites for reflective thinking are being met or not.
- To design tools and strategies that help students stay engaged, curious, and critical while using AI.
Let us now explore a few ways we can apply this framework of reflective thinking.
1. Encouraging Curiosity
The first key component for reflective thinking is a sense of perplexity—that moment of confusion or curiosity that makes you ask, “Wait, how does this work?” But when AI instantly gives us polished, confident answers, we might miss the chance to sit with our confusion and explore it.
One solution to this is designing learning approaches that invite learners to slow down and think. For instance, while interacting with GenAI tools, students could be enabled to highlight parts of an AI response that confuse or interest them. This not only encourages active engagement but could also give the AI some feedback to tailor future responses to support the students’ thinking. Another helpful strategy is to add a bit of “friction” to the learning process—small steps that require students to pause, reflect, or interact with the AI’s answer before they can use it.
2. Constructing Deep Knowledge
Another prerequisite of reflective thinking is persistent inquiry—digging deeper into ideas instead of just skimming the surface. But AI tools, such as chatbots, may tend to stay at the surface level, especially when users don’t know the right questions to ask or how to guide the conversation.
This is where schema theory can be helpful. Based on this theory, our brains organize knowledge like mental maps, or schemas, that help us connect new information to what we already know. We can build educational tools that make these mental maps visible. For instance, this could be implemented using interactive knowledge graphs that link ideas together. An example tool could allow learners to ask a question, based on which related concepts in the knowledge graph light up when the response is generated, helping them connect the dots between what they are learning and what they already know.
3. Promoting Thoughtful Reflection
GenAI tools often present answers with confidence, even when they’re wrong. Combined with their tendency to be agreeable, they may reinforce what we already believe. That’s a problem when it comes to being open to uncertainty during exploration, which is essential for reflective thinking.
To make students’ interactions with GenAI tools more active and thoughtful, we can use metacognitive prompts—well-timed questions or nudges that encourage learners to pause and think. For example, such a prompt might ask: “What’s another way to look at this?” or “What do you think about the AI response? Did you find anything surprising?” These gentle nudges could help students become more aware of their own thinking, especially in moments when they might otherwise accept AI output at face value.
To make students’ interactions with GenAI tools more active and thoughtful, we can use metacognitive prompts—well-timed questions or nudges that encourage learners to pause and think.
Moving Forward
If educators want students to grow into curious, capable thinkers, they need to design learning environments that invite them to do just that. That means creating space for confusion, encouraging deeper reflection, and guiding students to become more aware of how they think and learn. GenAI can absolutely be part of that journey, but only if it is used intentionally. Overall, what is most important to protect students’ thinking and learning is building habits of mind—curiosity, reflection, skepticism, and persistence—that ensure their thinking doesn’t get left behind in a world where AI is an integral part of our lives.
Read our recent paper on this topic: “Protecting Human Cognition in the Age of AI”.
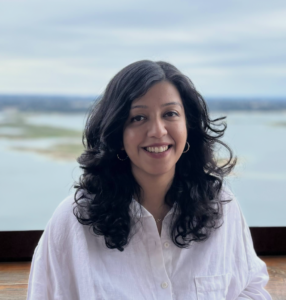