ChatGPT has become a popular tool for teachers in the classroom. For instance, a quick search for “ChatGPT essay scoring” will lead to plenty of guides and studies embracing the AI application. However, could using ChatGPT in the classroom for this purpose potentially harm students? How can we be sure the chatbot is fair with its scoring?
Research on fairness in ChatGPT’s essay evaluation capabilities is quite limited. So far, only two studies have looked into whether the chatbot favors certain groups of students (e.g., white students) over others (e.g., Black or low-income students). One study uncovered ChatGPT’s potential racial or ethnic bias in scoring: students described as Black or White were scored higher than those with race-neutral or Hispanic descriptions. However, with only a few studies available, the findings are inconclusive. Additional research is needed to deeply explore biases in ChatGPT’s use as a writing evaluation tool and to ensure both teachers and students are safely using it.
Thus, we assessed ChatGPT 4o for potential evaluation bias using the Automated Student Assessment Prize (ASAP) 2.0 benchmark. This benchmark is a publicly available dataset of around 24,000 argumentative essays from U.S. middle and high school students that our team collected. These essays reflect current standards in standardized writing assessments, and expert human raters scored them.
The dataset includes student demographic information like race/ethnicity, English Language Learner (ELL) status, economic disadvantage, and gender to facilitate fairness evaluations. In our analysis, we examined whether ChatGPT scores essays similarly to humans overall and across student race/ethnicity, gender, ELL status, or socioeconomic groups. We sought to address potential limitations in ChatGPT’s essay scoring, such as performance differentiation and bias.
Overall, the results show ChatGPT struggles in essay scoring, particularly in performance differentiation. The chatbot was less likely to distinguish high- and low-quality essays, assigning more scores near the average.
Overall, the results show ChatGPT struggles in essay scoring, particularly in performance differentiation. The chatbot was less likely to distinguish high- and low-quality essays, assigning more scores near the average (see Figure 1). Put differently, humans are likely to give students Fs and As. ChatGPT was more likely to give lots of Cs.
And while demographic bias was minimal, ChatGPT did assign lower scores to Black/African American than Asian/Pacific Islander students. Specifically, there was a moderate difference in scores between Black/African American and Asian/Pacific Islander students. However, there were no meaningful differences across the ELL status, gender, or socioeconomic group comparisons.
Methods For Assessing Bias In ChatGPT
The following analyses address the question, “Is ChatGPT biased?” and are based solely on the scores provided by ChatGPT 4o. To assess ChatGPT’s potential bias on the ASAP 2.0 benchmark, the chatbot was asked to assign holistic scores to the benchmark’s evaluation set of roughly 5,200 essays. These essays are not part of the publicly available dataset, which helps avoid data contamination (i.e., the chatbot may already know the score to assign due to prior exposure).
Members of the Learning Agency team, along with researcher Scott Crossley at Vanderbilt University, developed the ASAP 2.0 benchmark to provide the largest open-access dataset of scored student argumentative writing. The team designed the dataset to match U.S. national demographic details and mitigate the potential of algorithmic bias. Human raters evaluated each essay for holistic quality on a scale of 1 to 6. Although measures were taken to ensure fairness in the human ratings, such as anti-bias training among the evaluators, score differences did emerge across racial groups, such as slightly higher scores for Asian/Pacific Islander students compared to Black/African American students (see Table 1). However, these differences may not be due to rater bias alone but instead may reflect real-world disparities and inequities impacting Black/African American students in the U.S. public schooling system. Thus, our analytical focus was on whether ChatGPT would score different groups differently.
ChatGPT was set up using OpenAI’s standard API settings with a few-shot prompting method. The chatbot was given instructions, a sample essay for each score, and the percentage of essays receiving each score to enhance its performance (see Appendix 2 for an example prompt). This approach was designed to mimic how teachers might use ChatGPT in real-world scenarios while also testing how well the default version would perform without any sophisticated engineering.
ChatGPT gave a different range of holistic scores than human raters on the ASAP 2.0 benchmark. ChatGPT scored more essays as 3 or 4 and, interestingly, did not award any essays with a 6, whereas humans did provide essays with this high-achieving score.
Bias was evaluated using ANOVA (a test used to determine significant differences between means across more than two groups) and Welch’s T-test (a test used to determine significant differences between means across two groups). These tests indicate whether results are statistically significant, but they only tell part of the story. To understand the real-world importance of any observed score difference across groups, both statistical significance and practical significance were considered. Put differently, differences that are statistically significant (i.e., not due to random chance) may not be practically significant (i.e., effectual in the real world). We relied on p-values to indicate whether a result is statistically significant, and we relied on effect sizes to measure the magnitude or practical significance of the differences for real-world applications.
For instance, imagine you’re a teacher assessing two different classrooms’ test scores. Statistical significance, indicated by p-values, will help determine whether there’s any difference between the averages of the two classrooms, and ensure this difference is not due to random chance. Practical significance, indicated by effect sizes, determines whether the differences are large enough to impact the way you approach teaching each classroom. A statistically significant difference of .5 points may not influence your instructional choices, but a practical difference of 15 points likely would.
In the analyses below, practical significance is defined by a medium or larger Cohen’s d effect size (d = 0.5). This approach ensures the findings are mathematically meaningful for real-world decision-making. Other researchers have suggested an amendment in the interpretation of Cohen’s d effect size values, reducing the threshold for younger students. However, since our analyses are a case study on late middle school and high school student essays, Cohen’s original interpretation of effect size is used.
Findings
ChatGPT gave a different range of holistic scores than human raters on the ASAP 2.0 benchmark. As shown in Figure 1, ChatGPT scored more essays as 3 or 4 and, interestingly, did not award any essays with a 6, whereas humans did provide essays with this high-achieving score.
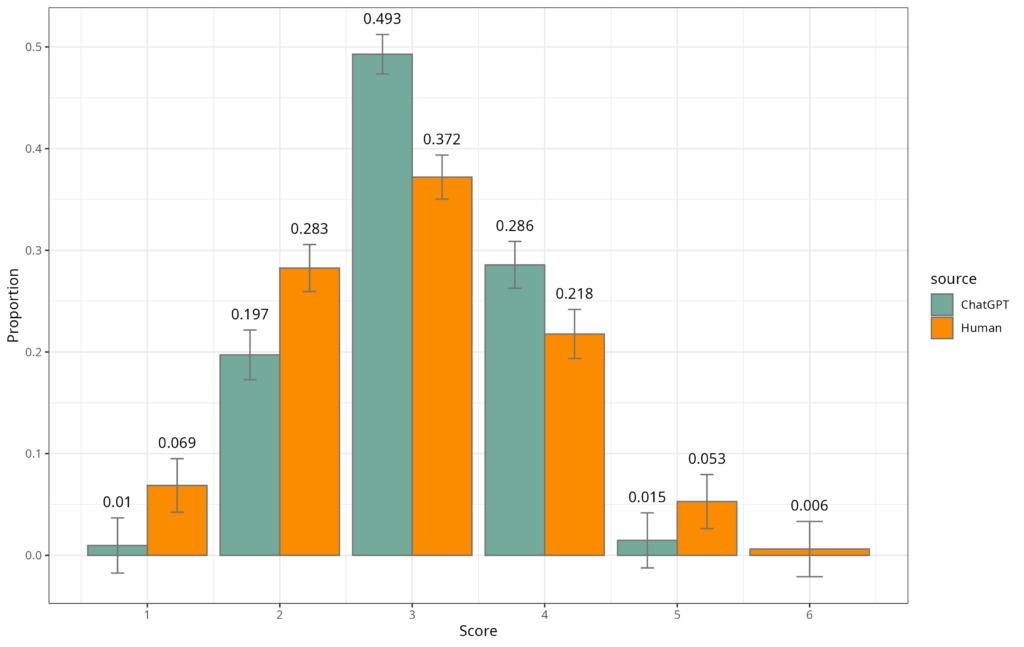
ChatGPT’s scores were further assessed by student subgroups of race/ethnicity, ELL status, economic disadvantage, and gender.
Race/Ethnicity
Overall, the average scores across different racial/ethnic groups were relatively close (see Figure 2), but some interesting patterns emerged. An ANOVA test revealed that the differences in scores were statistically significant (F = 25.61, p < 0.001). However, when we looked at the strength of this difference, the effect size (eta² = 0.024) was small, indicating a lack of practical significance.
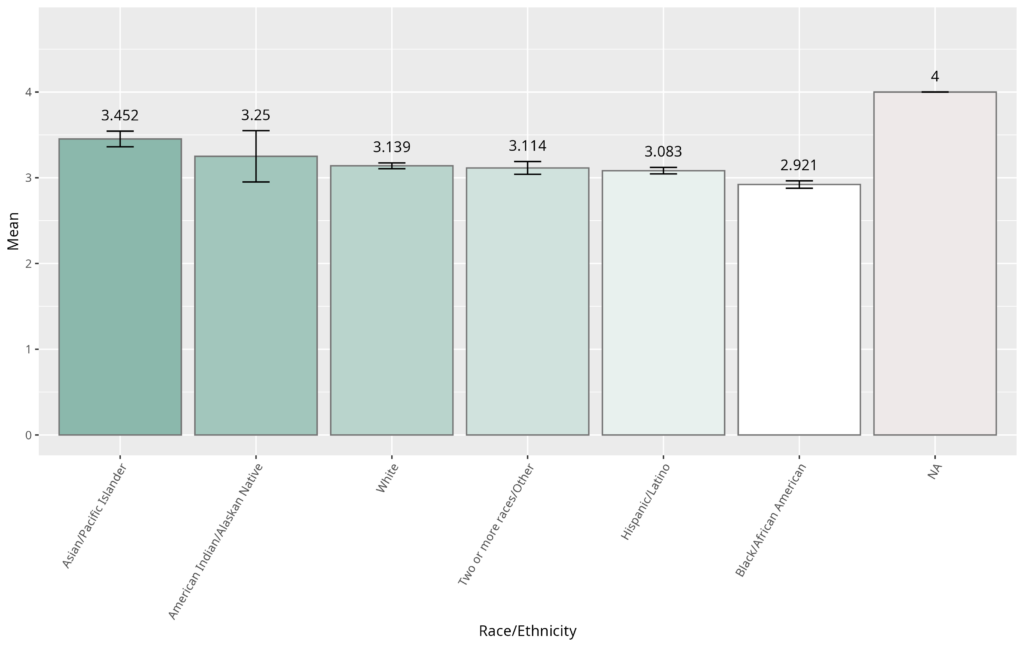
To dig deeper, Tukey tests were used to identify which racial/ethnic groups significantly differed (see Appendix 1). The results showed some significant differences between groups. Still, the effect size, measured by Cohen’s d, was small for all statistically significant differences except when comparing Black/African American and Asian/Pacific Islander students.
A medium effect size (d = 0.714) was found between Black/African American and Asian/Pacific Islander students. Thus, both a statistically and practically significant difference was found between these two groups. This result is consistent with the scoring differences observed among the expert raters, as described in the Methods section above. In other words, Black/African American performed slightly worse than Asian/Pacific students in writing the essays. This fact is observed in many other national assessments. The practical implications are that if both the chatbot and trained raters assigned lower scores to Black students than to Asian students, further analysis is needed to determine whether this disparity stems from rating bias or reflects real-world performance differences.
Moreover, the standard deviation of scores for each racial group is larger in human ratings than in ChatGPT’s ratings (see Table 1), though this was not analyzed using statistical tests. The increased standard deviations in human scores indicate wider variability in scoring than ChatGPT, suggesting the human ratings provided more meaningful differentiation in performance quality. This finding aligns with the different score distributions observed in Figure 1.
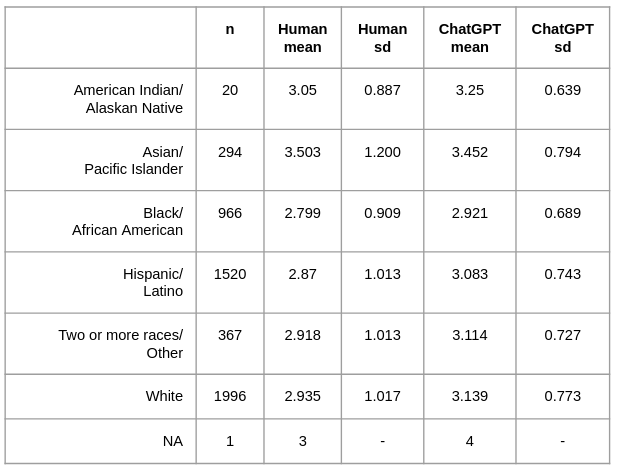
ELL Status
Similar to race/ethnicity, average ChatGPT scores across ELL status were relatively similar (see Figure 3). Welch’s T-Test revealed a statistically significant difference in scores (t = 8.205, p < 0.001), but the effect size, measured by Cohen’s d, was small (d = 0.337), indicating a lack of practical significance. These results align with the findings drawn from the analyses of the human scores.
Similar to the race/ethnicity findings, though not analyzed through statistical tests, the standard deviations of scores for each ELL group are larger in human ratings than in ChatGPT’s set (see Table 2), indicating greater variability in human scores, consistent with the different score distributions shown in Figure 1.
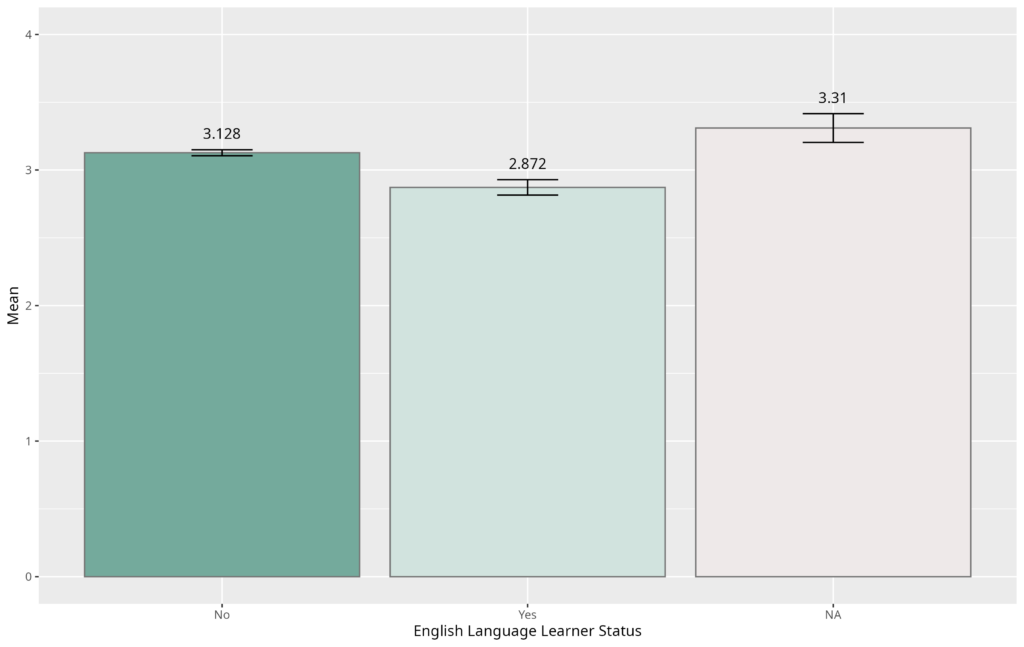
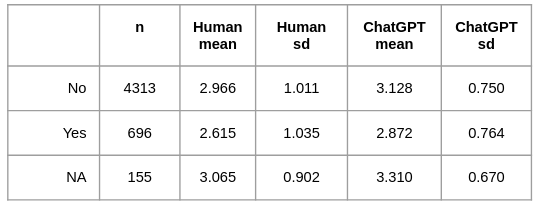
Economic Disadvantage
Average scores across economic disadvantage were also relatively close (see Figure 4).
Welch’s T-test found a statistically significant difference in scores (t = -13.111,
p < 0.001). Nonetheless, the effect size, measured by Cohen’s d, was small (d = -0.375), suggesting a lack of practical significance. These results align with conclusions observed in human scores with these analyses.
Though not analyzed through statistical tests, the standard deviations for scores in each economic disadvantage group are larger for the human ratings than ChatGPT’s ratings (see Table 3). The increased standard deviations in human scores indicate wider variability than ChatGPT scores, aligning with the different score distributions shown in Figure 1.
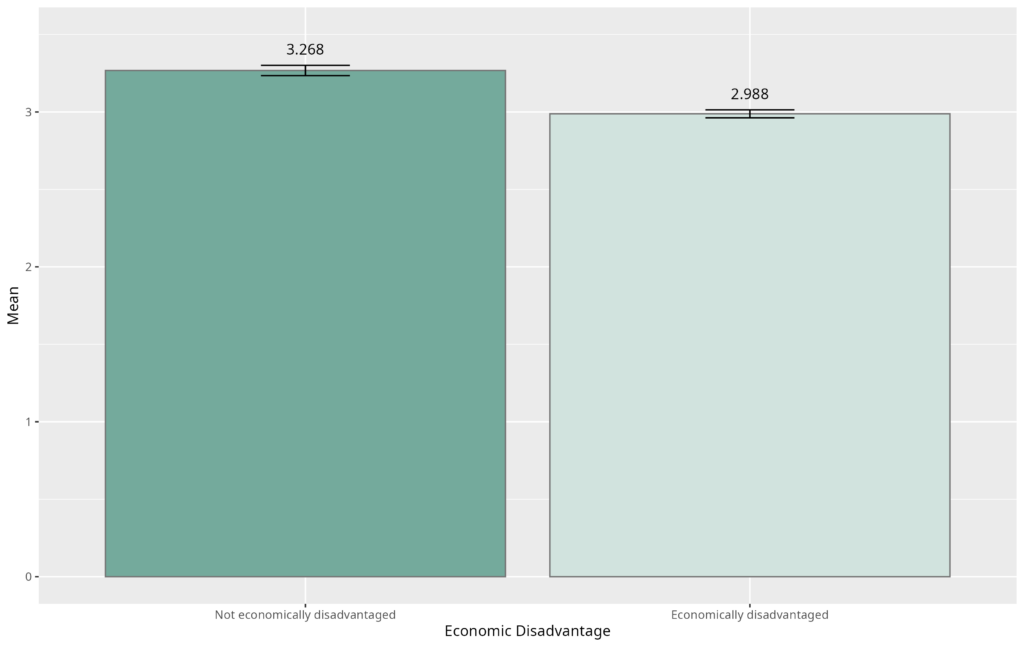
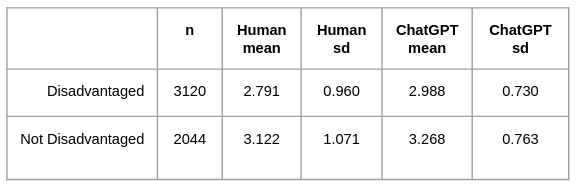
Gender
Lastly, average scores across gender were similar. Welch’s t-test found a statistically significant difference in scores (t = 2.787, p = 0.005). However, the effect size, measured by Cohen’s d, was small (d = 0.078), indicating a lack of practical significance. These results are consistent with findings from human scores in our other analyses.
Though not analyzed through statistical tests, the standard deviations of scores for each gender are larger in human ratings than in ChatGPT’s (see Table 4). The increased standard deviations in human scores indicate greater variability compared to ChatGPT’s scores, aligning with the different score distributions shown in Figure 1.
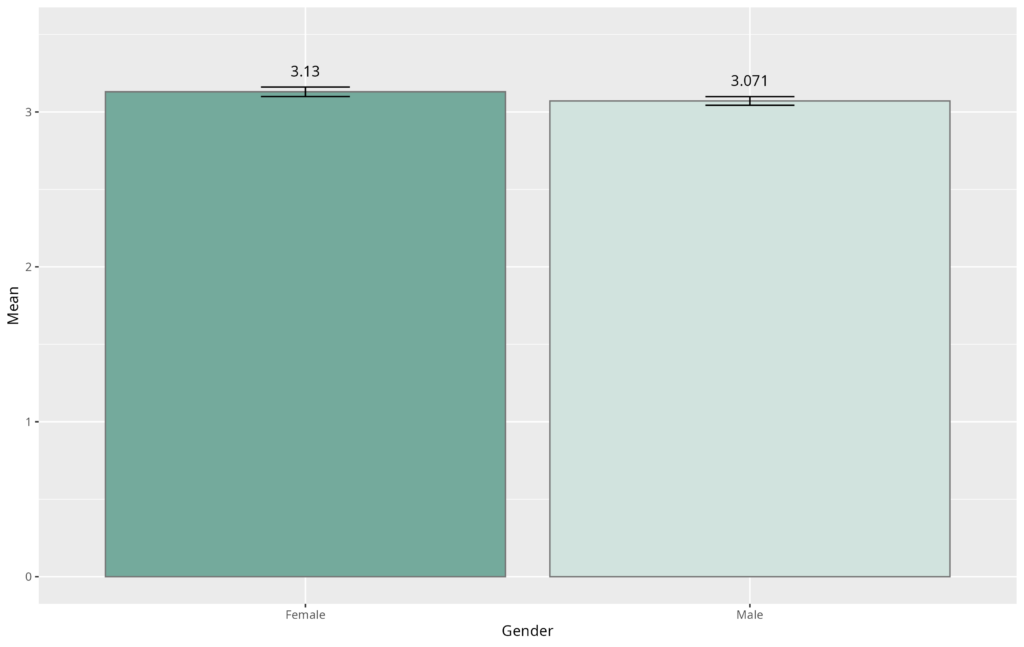
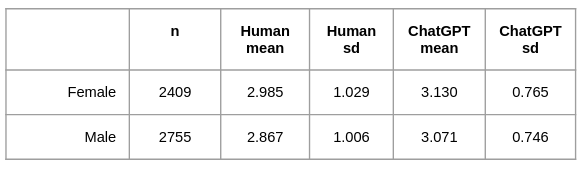
Additional Analyses
We explored other methods to assess fairness in ChatGPT’s essay scoring, but our findings were consistent: minimal bias.
The first analysis was a one-sample t-test to compare the average ChatGPT score for a specific student group to the chatbot’s average score across all groups (x̄ = 3.10; Appendix 3). Note that p-values were adjusted using the Benjamini and Hochberg FDR to account for increased false positive rates, considering the number of tests performed. The results revealed statistically significant differences for Asian/Pacific Islander, Black/African American, White, economically disadvantaged, and non-disadvantaged students. However, the effect sizes were small or negligible for all groups as measured by Cohen’s d, meaning the differences were not practically significant. In other words, ChatGPT’s average score for a subgroup was similar to ChatGPT’s average score for all students.
The second analysis was a pairwise t-test to compare the average ChatGPT score to the average human score for each demographic group (Appendix 4). The results showed statistically significant differences between ChatGPT and human scores for all demographic groups besides American Indian/Alaskan Native and Asian/Pacific Islanders. However, all Cohen’s d effect sizes were small or negligible, showing no practical significance. In other words, ChatGPT’s average score for each student group was fairly similar to the average human score for that respective group.
These findings show that ChatGPT’s scoring is consistent across student groups in terms of average scores. However, as noted in our previous analyses, the chatbot struggles to distinguish low- and high-quality essays as effectively as humans.
Discussion
Overall, these findings reveal that ChatGPT’s scoring bias was minimal, but a greater performance issue may lie in its ability to properly distinguish essay quality. The chatbot was more likely to assign scores near the average, reducing meaningful differentiation in essays (see Figure 1). While ChatGPT gave more 3s and 4s, humans gave more 1s, 2s, 5s, and 6s. This slight variation is further seen in subgroup analyses across ELL, race/ethnicity, gender, and socioeconomic categories (Appendix 4).
If one limitation in ChatGPT’s scoring is its low variability, then one implication is that researchers can’t rely on mean differences alone to compare human- and machine-generated scores. While ChatGPT may not be biased in the traditional sense, if it gives all students a similar score, that’s a bigger concern. If the chatbot can’t distinguish between high- and low-performing writers, the tool may not be optimal for automated essay scoring because it can’t meaningfully differentiate student performance.
Additionally, if historically underperforming populations are not receiving high scores—scores they would get from a human teacher—that could still render the system unfair, as vulnerable students may not receive the credit they deserve in an AI system.
Overall, these findings reveal that ChatGPT’s scoring bias was minimal, but a greater performance issue may lie in its ability to properly distinguish essay quality. The chatbot was more likely to assign scores near the average, reducing meaningful differentiation in essays. While ChatGPT gave more 3s and 4s, humans gave more 1s, 2s, 5s, and 6s.
When our team explored potential bias in ChatGPT’s scoring regarding race and ethnicity, we observed that ChatGPT assigned lower scores to Black students than to Asian students. However, this pattern mirrored the human-provided scoring differences in the ASAP 2.0 benchmark and the national assessment data on writing outcomes for Black and Asian students. Thus, another approach could involve controlling for student performance – ensuring that scores between Black and Asian students are compared when the underlying quality of work is equivalent – to determine whether ChatGPT exhibits or introduces new bias.
It’s also important to note that this study only looks at ChatGPT’s bias in grading argumentative essays written by middle and high school students. ChatGPT might show greater bias when assessing students in other grade levels, writing tasks, or evaluation tasks (e.g., providing generative feedback). Thus, teachers using ChatGPT or any AI tool must broadly assess its use to ensure it’s suitable for their classroom.
Additional approaches can be taken to explore bias in large language models (LLMs) like ChatGPT. For instance, engineers could fine-tune an LLM to grade essays for a specific population, and then test the model’s performance on essays from other populations. However, this approach, along with others, is impossible without robust student demographic details and metadata to conduct the fairness evaluation. Student metadata is a key feature of the ASAP 2.0 benchmark, providing comprehensive details on a student’s demographic background.
Thus, high-quality benchmarks like ASAP 2.0 are essential to ensuring fairness and integrity in chatbot tools. Education datasets are too rarely released for open research and development, yet alone data with robust learner profiles. However, they are fundamental to developing fair and accurate AI models. Enhancing AI’s safety in the classroom will require advancing the work of AI benchmarking and releasing robust datasets representing diverse student populations and learning contexts.
Appendix 1: Tukey Test Results
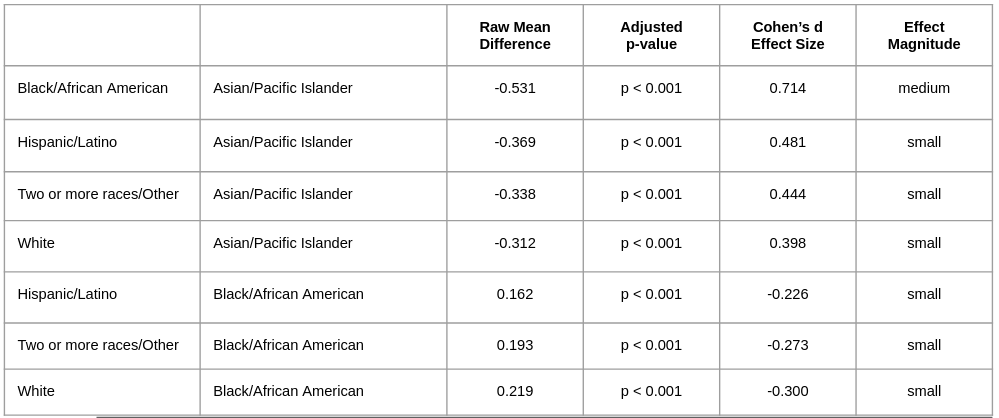
Appendix 2
Note: This appendix provides an example of few-shot and percentage prompting for a score of 6. Example prompts and percentages for each score (1 to 6) were provided to ChatGPT when asking it to provide holistic scores to the ASAP 2.0 benchmark to improve performance.
ASAP 2.0 Few Shot + Percentage Prompting
prompt = “””You will be provided with an argumentative essay produced by a 6th-12th grade student in the United States for 1 of 15 prompts. After reading the single essay, assign a holistic score based on the rubric below. For the following evaluations you will need to use a grading scale between 1 (minimum) and 6 (maximum). As with the analytical rating form, the distance between each grade (e.g., 1-2, 3-4, 4-5) should be considered equal.
[SCORE OF 6: An essay in this category demonstrates clear and consistent mastery, although it may have a few minor errors. 0.61% of all essays received a SCORE OF 6]
An example essay with a SCORE OF 6 is as follows:
In “The Challenge of Exploring Venus,” the author suggests that studying Venus is a worthy pursuit despite the dangers it presents. The author has a thorough argument; however, he makes his point slightly confusing when he introduces irrelevant and unclear topics, uses informal language, and bounces between topics of supporting and not supporting the concept. I do not think the author supports their idea thoroughly due to this and the other factors.
Primarily, the author’s points are very unclear and are irrelevant to his proclaimed position in the beggining paragraphs. He begins to dive into the topic of Venus and its name origins. He discusses the relation of Venus to Earth, but does not specifically say how this correlates to exploring Venus. The author should have included a strong thesis instead of making it very misleading when discussing Venus’s nickname. In Paragraph 2, he slightly expands on the exploration of Venus. However, when he does so, he discusses the difficulties of accomplishing this and not how this will be a valued exploration. This in no way supports his proposal. In Paragraph 4, he reintroduces Venus’s features and how they are analogous to Earth as well as how it can be “our nearest option for a planetary visit”. Although he does show some correlation to his point through this, it is still not very relevant and does not show how worthy of a pursuit it will be of studying Venus.
Additionaly, the author uses informal rhetorical questions and language that counter his argument. In Paragraph 4, he states “If our sister planet is so inhospitable, why are scientists even discussing further visits to its surface?” In a way, this could have supported his argument due to the scientists appearing to want the exploration, but the author asks it in a question form that contradict it otherwise. The author proceeds to answer the question but he utilizes informal language such as “probably” that make his point appear unstable. He continues to explain how similarly Earth and Venus are and finally includes how exploring Venus can be beneficial. He then concludes the paragraph with another question, inquiring about how to make the journey safe and productive. The author should have asked the rhetorical questions in a more suitable way that support his argument. With the concluding question, he somehwat sounds in favor of the exploration, but he still appears unsure because he is not aware of the options “for making such a mission both safe and sceintifically productive”. Furthermore, the author continuously uses words such as “maybe” and personal pronouns that sound very informal. In a way the personal pronouns relate to the reader. However, when utilizing the pronoun “our”, it’s very confusing to the argument when used in the first statement in Paragraph 4. The author says “our sister planet” which can sound like the author is asking for support against the idea through the use of pathos.
Finally, the author always appears to be countering his own argument. For example, in paragraph 6, he states, “peering at Venus from a ship orbiting or hovering safely far above the planet can provide only limited insight on ground conditions…”. This can be interpreted as the author not being satisfied by the progress that will be made and is expecting something more progressive. The author should have demonstrated some support towards the idea instead of degrading it. He proceeds to be unsupportive when he discusses how the scientists are unable to take samples from Venus from a distance. If the work is progressive and is more beneficial than it was several years ago, the author should discuss how they could improve their missions or at least demonstrate some supportiveness of the progress. In addition to countering his own argument, the author repeatedly stated how toxic the environment was on Venus that it was nearly impossible to sustain human life or have any form inhabit it. He goes into great detail about how lethal Venus’s atmosphere is in Paragraph 3. He states “these conditions are far more extreme than anything humans encounter on Earth”. Although the author does include how danger the exploration might be, he does not discuss how it will be a worthy pursuit.
In conclusion, I do not think the author supports their idea that studying Venus is a worthy pursuit despite the dangers. The argument could have been well-supported but it was not. Instead, it was contradicted numerous times and was pushed away by irrelevant topics.
[Final Instructions]
You will be provided with a single essay written by a student. Respond with your score in the following format: SCORE OF X. Make sure that you follow the score format SCORE OF X.
Appendix 3
One-sample t-test results comparing the ChatGPT demographic group mean to the ChatGPT Overall Mean (x̄ = 3.10).
*Note: P-values are adjusted using Benjamini and Hochberg FDR in each family of tests to account for inflation of false positive rate
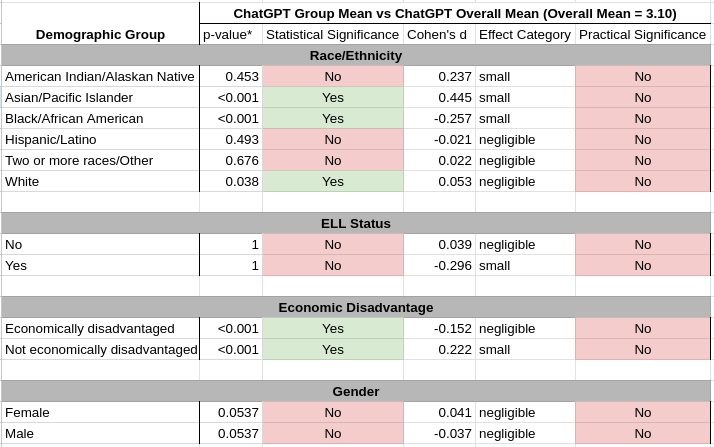
Appendix 4
Paired t-test results comparing the ChatGPT demographic group mean score to the Human demographic group mean score.
*Note: P-values are adjusted using Benjamini and Hochberg FDR in each family of tests to account for inflation of false positive rate
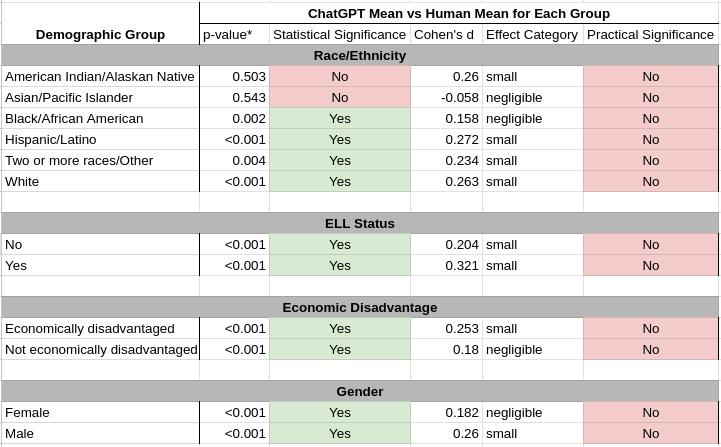
This article was written by The Learning Agency staff members L Burleigh, Kennedy Smith, Perpetual Baffour, Ulrich Boser, and Vanderbilt University researcher Langdon Holmes.
2 thoughts on “Identifying Limitations and Bias in ChatGPT Essay Scores: Insights from Benchmark Data”
Thank you for sharing this research. In order to better understand the results, I’d like to see the rubric that was used to score essays holistically. Is that something you can share?
Bar charts and histograms are not particularly helpful for comparing distributions, since the greater number of extreme values for human scorers inevitably means there will be fewer central values. A much better way to get a feeling for the data is to use cumulative frequency charts (and, ideally, reverse cumulative frequency charts, so that the higher graph represents higher performance). Also, the analysis assumes that the humans are right. Some recent studies have found that LLMs are better at sticking to the rubric than human scorers are. In response, people say that humans are recognizing aspects of quality that were not captured by the rubric, but then is it fair for teachers to give students a rubric, but then to use other factors that are not disclosed to students in scoring?