The Learning Engineering Virtual Institute, or LEVI, is a collaboration of researchers, learning engineers, and educators striving to develop, scale, and implement new tutoring platforms that can double the rate of math progress among middle school students, especially those from low-income backgrounds. Since 2022, seven teams have been working to achieve this goal. Their ideas range from an AI-powered chatbot that provides personalized math tutoring to AI video technology that digitally replicates the experience of having a personal tutoring session.
MATHstream, from Carnegie Learning, is an adaptive, interactive video streaming platform designed for middle and high school students. Far from passive, each lesson is led by an engaging math influencer who brings concepts to life through a dynamic, game-based approach. The platform responds to students in real time, encouraging them to apply new skills, push their limits, and stay motivated. At the same time, teachers can seamlessly monitor progress, making MATHstream a powerful tool for both learning and instruction.
In this installment of “5 Questions,” Jamie Sterling of Carnegie Learning talks about the team’s work and discoveries.
What was your “ah-ha” moment when you knew you were onto something workable?
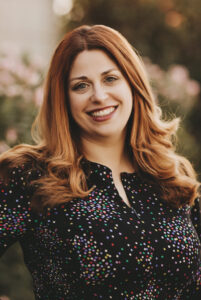
Our “ah-ha” moment was not a single thing, but was a combination of pivotal experiences that largely centered on user feedback and/or co-design sessions that highlighted the impact and potential of our work. Feedback from both students and teachers has consistently reflected a “wow factor,” validating our efforts. Each of these moments contributed to our realization that we have something truly transformative on our hands.
The first came from the students themselves; their excitement and engagement, especially during visits from MATHStreamers and during our user testing. Seeing this enthusiasm firsthand solidified our confidence that we were onto something powerful.
The second observation was from implementation – as school districts began creatively using MATHStream in ways we hadn’t initially considered, it reinforced that the tool was versatile and adaptable, but also that we needed to tell the implementation story more clearly.
A surprising validation came during a Prolific study through the Engagement Hub, where we tested engagement metrics with an adult participant who had previously struggled with math. She shared that watching our videos made her understand mathematical concepts for the first time. That breakthrough, along with high engagement metrics from the study, showed us that our content could resonate deeply with learners beyond our initial target audience.
A final recent, but major “aha” moment for the team came when we successfully prototyped streaming avatars to provide real-time video hints, which made our long-term vision feel tangible and achievable.
Have you made any significant shifts or course corrections?
Yes, we’ve made several important shifts while working with LEVI, largely driven by the fast-paced advancements in AI technology. Early on, we planned for gradual improvements, but AI’s rapid evolution opened new possibilities for accelerated testing – like real-time video response generation and expanded interaction modalities. This pushed us to explore ways to implement these capabilities sooner, while also considering the scalability of moving from research to product, including pricing strategies, resource needs, and potential use cases.
We’ve also adjusted our approach to generative AI, particularly to build trust with users and validate the platform’s effectiveness. This means temporarily slowing down certain developments to ensure we’re creating a reliable foundation, especially as we continue learning from our prototypes, such as the video-based streaming avatar. These experiences are helping us plan a smoother path from pilot to scalable product.
Additionally, we’ve begun focusing more on how student agency shapes our platform’s design and development. While accommodating all preferences is not feasible, we’re zeroing in on what really matters to students to enhance their learning experience. Originally, our vision centered on adaptive, LLM-driven segments. Now, we’re leaning towards on-demand dialogic support at each learning step, made possible through LiveHintAI and HAILP, which allow us to provide timely, personalized feedback.
Generative AI’s quick progress has led us to accelerate proof-of-concept work for these tools. Although these shifts weren’t in our original plan, they are helping us move closer to delivering impactful, learner-centered experiences.
What’s been the most surprising thing your team has learned?
From a more positive perspective, one insight we’ve gained so far is the importance of character design in AI-based learning agents. Our initial theory was that relatability would hinge on making these characters as realistic as possible, yet we found that even non-realistic, cartoon-like characters can be engaging and relatable for students. This has reshaped our approach to developing AI agents, especially in balancing visual design with functionality and trust-building. Another important takeaway around trust is that students generally understand what an AI bot is and appreciate when there is an introduction to the bot and where it lacks humanity.
From a learning science and more negative perspective, we were surprised there weren’t more noticeable impacts when we tested the video rewind button. Although adding a rewind button was one of the most requested features, it didn’t significantly impact student performance on assessments. While the feature remains valuable from a usability perspective, we expected to see a more measurable effect on learning outcomes, challenging some assumptions about how students interact with video content.
These insights are helping us refine both our product and our understanding of how students engage with AI-driven learning tools.
When did you see MATHstream working for students and teachers?
One really interesting use case that a partner shared with us was the implementation of MATHstream in a classroom for students new to the country. In this classroom, students speak many different languages. The teacher paired up students who spoke the same language and had them work together on MATHstream. The teacher wanted the videos in English but encouraged students to rewind frequently and to talk through the problems, which helped with both their math and their English.
We visited multiple partner schools during this project, which always provides a great opportunity to gain a deeper understanding of how MATHStream is being used. Students are generally intrigued by the engaging video content and gamified elements and are eager to provide their feedback. Students also think our MATHstreamers are really engaging (and based on our use of them on social media, where their posts exceed our average engagement, we believe teachers agree).
What do you anticipate your project accomplishing in 5 years?
In five years, we envision MATHStream and MATHia working together seamlessly to offer an even more dynamic and personalized learning experience. Leveraging advancements in AI, our goal is to move beyond text-based feedback to provide students with rich, real-time video and animated guidance that adapts to their individual problem-solving needs.
We see the potential for MATHStream to deliver conversational, data-driven support that empowers students to build confidence and improve accuracy, all while remaining cost-effective. Generative AI will play a significant role in delivering error-specific, targeted feedback, creating powerful “aha” moments that can transform understanding.
Additionally, we aim to provide robust professional learning guidance, continuously refining our understanding of how students and teachers interact with MATHStream so that we can identify and promote the most effective use cases. This will help teachers integrate our solutions fully and confidently into their classrooms.
Our vision includes establishing MATHStream as an adaptive component of math education, delivering personalized experiences personalized to students’ cognitive and, increasingly, non-cognitive needs. Ultimately, we also hope to leverage AI responsibly and contribute meaningfully to the broader dialogue on the ethics and impact of these technologies in education.
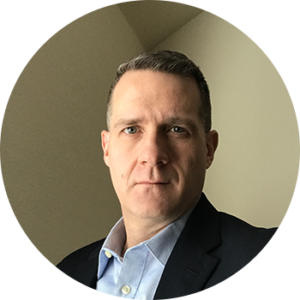